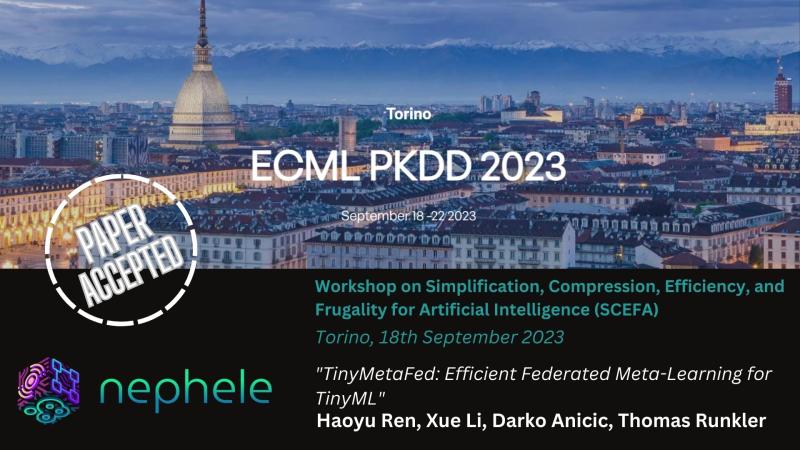
The European Conference on Machine Learning and Principles and Practice of Knowledge Discovery in Databases (ECML PKDD) is the flagship European machine learning and data mining conference. 2023 edition lasted for five days, from the 18th to the 22nd of September 2023. The conference main venue was OGR (Officine Grandi Riparazioni), the hub of innovation and art in Torino, Italy. The event was organized by Centai (Center for Artificial Intelligence) and Politecnico di Torino.
The conference attracts annually a worldwide audience of over 1000 attendees and is a unique opportunity for companies to develop connections in both machine learning and data mining communities, acquire new talents, contribute to innovation and market their brands. The conference web site received several tens of thousands of visits until the end of September 2023.
The conference program included presentations of peer-reviewed novel research, invited talks by leaders in the field, a wide program of workshops and tutorials, poster sessions, a discovery challenge, a demo track and an Applied Data Science track. It also featured an Industry Track, which will give companies an additional opportunity to advertise during the conference.
The Workshop on Simplification, Compression, Efficiency, and Frugality for Artificial Intelligence (SCEFA) was collocated with this event on 18th Sep 2023 in Room 3i of Politecnico di Torino, Italy. SCEFA focused on developing frugal AI models that can handle data efficiently, while minimizing energy consumption and environmental impact. As AI models continue to grow in complexity, their energy consumption and carbon footprint become more of a concern. SCEFA explored the challenges of training with limited data and computational resources, and examined approaches like pruning, quantization, and knowledge distillation to deploy energy-efficient models. The goal was to talk about the development of algorithms that reduce energy consumption while maintaining robust performance in the face of noise.
Workshop agenda:
9.00-9.05 Welcome from the organizers
9.05-10.00 Keynote: Florence D'Alché-Buc
10.00-11.00 Spotlights (5' each)
11.00-12.00 Coffee break + poster session
12.00-12.55 Keynote: Wojciech Samek
12.55-13.00 Concluding remarks
The paper “TinyMetaFed: Efficient Federated Meta-Learning for TinyML” by Haoyu Ren, Xue Li, Darko Anicic and Thomas Runkler with acknowledgente to NEPHELE funding was accepted by this workshop.
The field of Tiny Machine Learning (TinyML) has made substantial advancements in democratizing machine learning on lowfootprint devices, such as microcontrollers. The prevalence of these miniature devices raises the question of whether aggregating their knowledge can benefit TinyML applications. Federated meta-learning is a promising answer to this question, as it addresses the scarcity of labeled data and heterogeneous data distribution across devices in the real world.
However, deploying TinyML hardware faces unique resource constraints, making existing methods impractical due to energy, privacy, and communication limitations. We introduce TinyMetaFed, a model-agnostic metalearning framework suitable for TinyML. TinyMetaFed facilitates collaborative training of a neural network initialization that can be quickly fine-tuned on new devices. It offers communication savings and privacy protection through partial local reconstruction and Top-P% selective communication, computational efficiency via online learning, and robustness to client heterogeneity through few-shot learning. The evaluations on three TinyML use cases demonstrate that TinyMetaFed can significantly reduce energy consumption and communication overhead, accelerate convergence, and stabilize the training process.