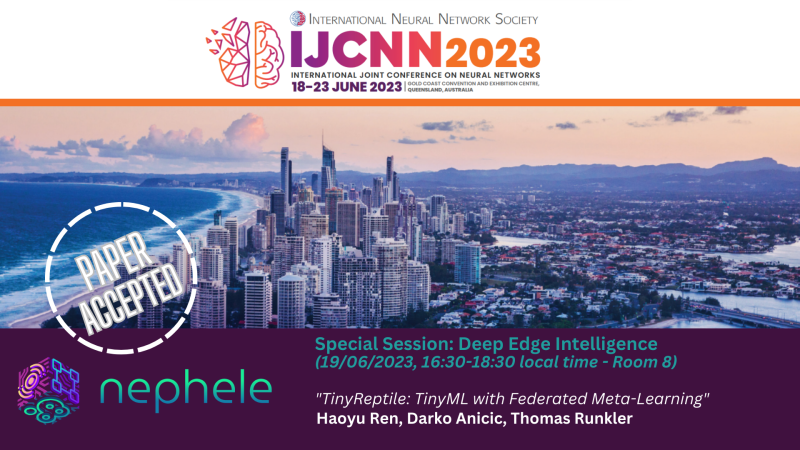
The International Join Conference on Neural Networks (IJCNN) is the biggest and the most prestigious forum to report on the new developments in neural networks, neuroinformatics and neuro-technologies, with over 1000 papers presented and published in the IEEEE IJCNN Proceedings. It is co-organised and co-sponsored by the International Neural Network Society (INNS) and the IEEE Computational Intelligence Society.
The paper “TinyReptile: TinyML with Federated Meta-Learning” by Haoyu Ren, Darko Anicic and Thomas Runkler from SIEMENS with acknowledgement to NEPHELE was presented in the Special Session “Deep Edge Intelligence” chaired by Kai Qin and Amit Trivedi, that took place in Room 8 at 16:30 – 18:30 (local time).
Tiny machine learning (TinyML) is a rapidly growing field aiming to democratize machine learning (ML) for resource-constrained microcontrollers (MCUs). Given the pervasiveness of these tiny devices, it is inherent to ask whether TinyML applications can benefit from aggregating their knowledge. Federated learning (FL) enables decentralized agents to jointly learn a global model without sharing sensitive local data. However, a common global model may not work for all devices due to the complexity of the actual deployment environment and the heterogeneity of the data available on each device. In addition, the deployment of TinyML hardware has significant computational and communication constraints, which traditional ML fails to address. Considering these challenges, this paper proposes TinyReptile, a simple but efficient algorithm inspired by meta-learning and online learning, to collaboratively learn a solid initialization for a neural network (NN) across tiny devices that can be quickly adapted to a new device with respect to its data. The team demonstrated TinyReptile on Raspberry Pi 4 and Cortex-M4 MCU with only 256-KB RAM. The evaluations on various TinyML use cases confirm a resource reduction and training time saving by at least two factors compared with baseline algorithms with comparable performance.