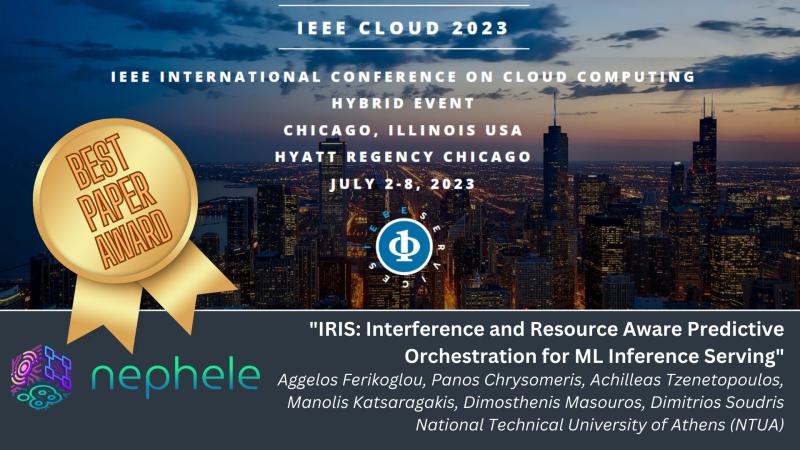
The paper IRIS: Interference and Resource Aware Predictive Orchestration for ML Inference Serving by our colleagues Aggelos Ferikoglou, Panos Chrysomeris, Achilleas Tzenetopoulos, Manolis Katsaragakis, Dimosthenis Masouros, and Dimitrios Soudris from National Technical University of Athens! - with acknowledgment to the NEPHELE project - has recently received the Best Paper Award in the IEEE International Conference on Cloud Computing (IEEE Cloud 2023).
Over the last years, the ever-growing number of Machine Learning (ML) and Artificial Intelligence (AI) applications deployed in the Cloud has led to high demands on the computing resources required for efficient processing. Multiple users deploy multiple applications on the same server node to maximize Quality of Service (QoS); however, this leads to increased interference. In addition, Cloud providers aim to minimize their operating costs by efficiently utilizing the available resources. These conflicting optimization goals form a complex paradigm where efficient scheduling is required. In this work, we present IRIS, an interference- and resource-aware predictive inference scheduling framework for ML infer-ence serving in the cloud. We target the multi-objective problem of QoS maximization with effective CPU utilization based on Queries per Second (QPS) predictions by proposing a modelless ML-based solution and integrating it into the Kubernetes platform.
This paper evaluates this approach over real hardware infrastructure and a set of ML applications. Its experimental analysis shows that under various QoS constraints, the model-specific interference-aware scheduler violates QoS constraints less frequently by achieving 1.8× fewer violations, on average, compared to over-provisioning and 3.1× fewer violations compared to under-provisioning, through efficient exploitation of available CPU resources. The model-less feature is able to cause, on average, 1.5× fewer violations compared to the model-specific scheduler, while further reducing the average CPU utilization by ≈ 30%.